Comparing Well and Geophysical Data for Temperature Monitoring Within a Bayesian Experimental Design Framework
Comparing Well and Geophysical Data for Temperature Monitoring Within a Bayesian Experimental Design Framework
Our new research paper was just published in Water Resources Research
We used Bayesian Evidential Learning (BEL) to optimize the design of a 4D temperature field monitoring experiment. We demonstrated how BEL can take into account various data source combinations (temperature logs combined with geophysical data) in the Bayesian optimal experimental design (BOED). To determine the optimal data source combination, we used the RMSE of the predicted target in the low dimensional latent space where BEL is solving the prediction problem.
The code used for this publication is, as always, open-source and can be found on GitHub.
The dataset and notebooks used to reproduce the results are also available on Kaggle.
If you are unable to access the paper, please contact me directly via email or ResearchGate
Additionally, we have a preprint available on EarthArXiv.
Anyone interested in learning more about the methods we propose or trying them out is always welcome to get in touch with us.
Key points
- We propose a method for optimizing the design of a four-dimensional (4D) temperature field monitoring experiment
- We use Bayesian Evidential Learning (BEL) to infer the posterior distribution of the temperature field for the experiment design
- We demonstrate how BEL can combine temperature logs and Electrical Resistivity Tomography data to assist in the design of experiments for a 4D temperature monitoring
Some illustrations
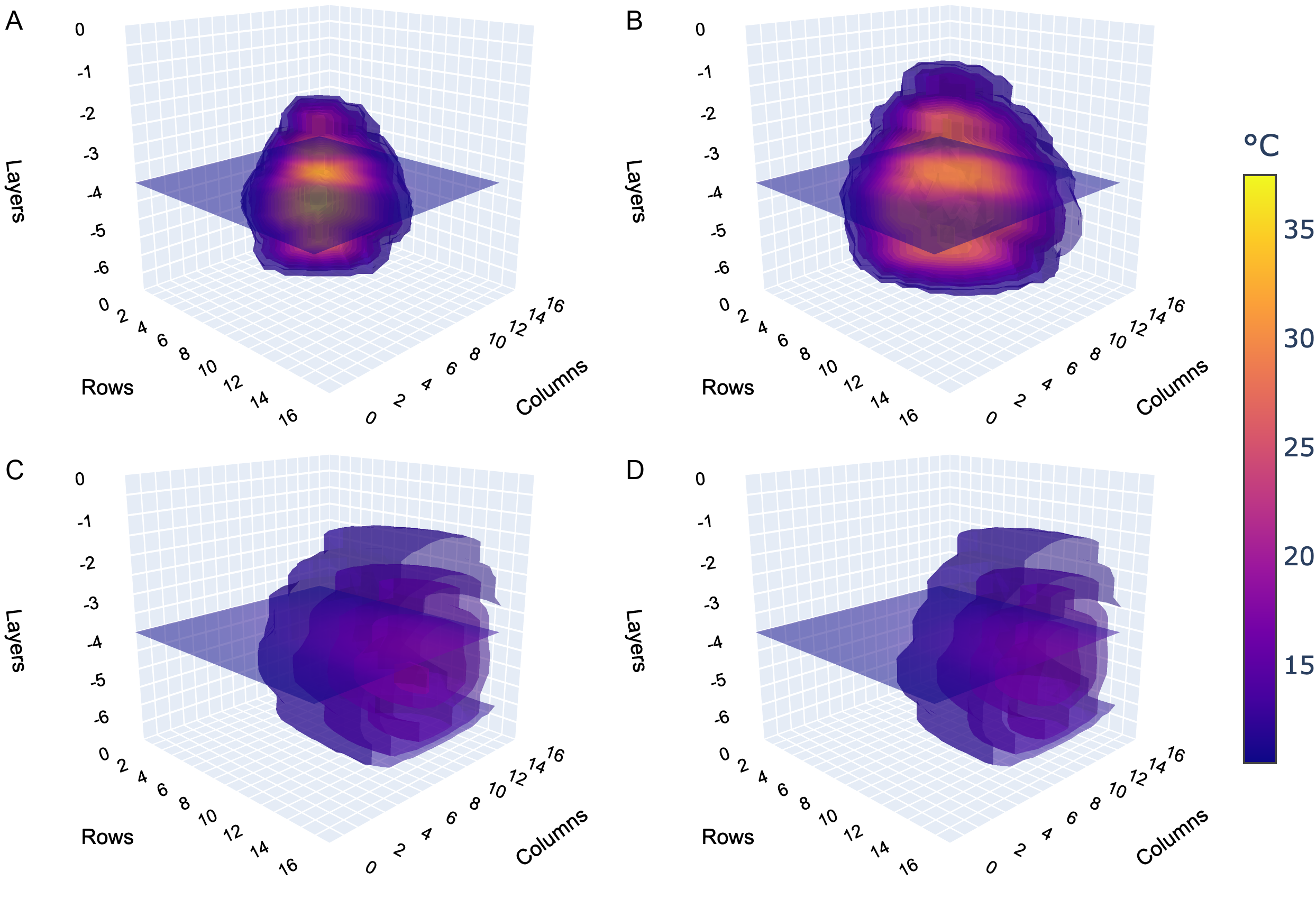
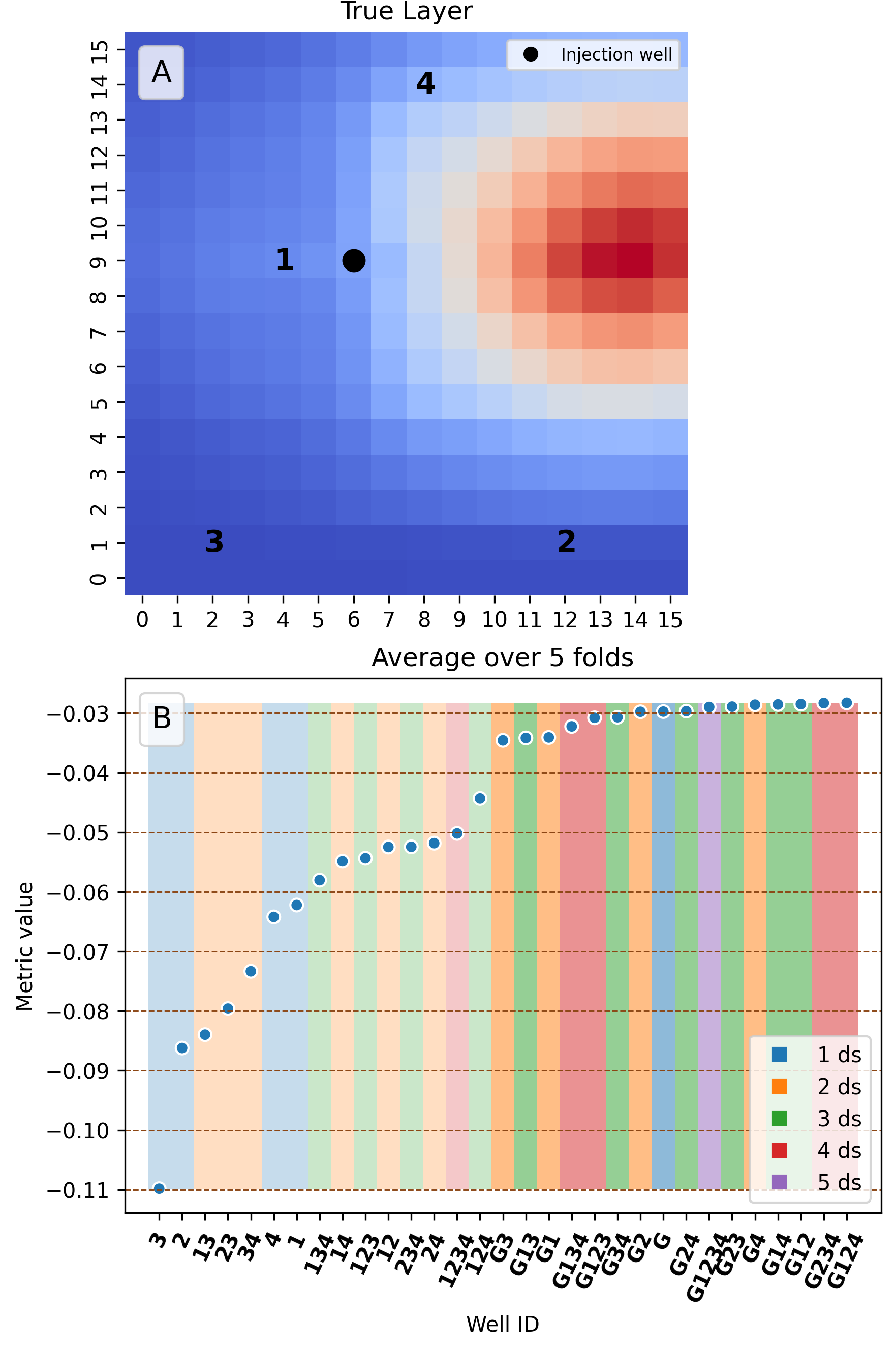